3 Considerations for Integrating Wearables into Sports Performance Monitoring
07/07/2022
Share this post
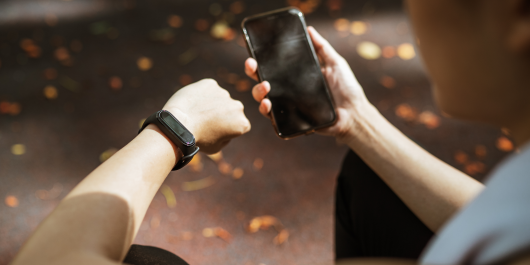
The past decades have witnessed an explosion of the development of wearable devices aimed at improving sport performance. Simultaneously, the excitement (and pressure) to integrate wearables as athlete monitoring tools has grown. Any sports scientist will tell you that sports performance is influenced by many factors – peak performance requires careful training prescription, effective recovery techniques, and proactive injury management, among other factors. The breadth of available wearables combined with the web of factors that influence sports performance makes it challenging for users to select the right tool for the job.
The purpose of this article is to discuss three considerations when using wearables to monitor factors associated with sports performance.
The goal is to empower sports scientists and athletes with takeaways for how to evaluate if a wearable is an accurate, actionable, and practical tool for monitoring sports performance.
3 Considerations for Integrating Wearables into Sports Performance Monitoring
#1: Sports performance wearables should be motivated by a signal of interest, not hardware availability.
Electrocardiogram signals, which provide information about the function of the heart, have been used as a clinical monitoring and diagnostic tool for over a century (circa 1880s [1]). The desire to monitor this clinically relevant signal outside the doctor’s office and during exercise motivated the invention of wearable heart rate monitors. Polar filed its first patent for a wireless heart rate monitor in 1977. In the following decades, the integration of wearable heart rate monitoring revolutionized endurance training. Decades of research have now demonstrated that heart rate and related measures (e.g., energy expenditure, maximal oxygen uptake, heart rate variability) provide measures of exercise intensity [2]. With this knowledge, athletes can use heart rate wearables to balance positive training adaptions while also looking for signs of overtraining. Of note, an ongoing challenge of heart rate monitoring is there are not definitive benchmarks for “optimal” training intensity (see consideration #2 below).
In summary: First there was a need to monitor heart rate during exercise (a signal of interest). Then, wearable heart rate monitors were developed (hardware availability). Finally, heart rate monitoring was integrated into sports performance monitoring using research for how the wearable signals should be interpreted. The integration of wearable heart rate monitors into sports performance monitoring provides an example of wearable usage that is motivated by a signal of interest.
As an alternative example, the miniaturization of accelerometer and inertial measurement unit (IMU) technology made it an exciting hardware option to integrate into sports performance monitoring (e.g., in running [3], skiing [4], basketball and soccer [5], etc.). Small, portable, and affordable IMUs became easy to integrate into activity monitors, watches, insoles, footwear, smart garments, and small sensor packages that could easily be clipped or strapped onto the body [6]. An example use case is wearable accelerometers that report body/leg/foot acceleration, impact, loading rate or shock as an indicator of running quality and risk of injury. It may feel instinctual that large running impacts are harmful for the body. However, running impacts are not equivalent to the loading and wear and tear experienced by musculoskeletal tissues at risk of injury [7], [8]. For example, the impact force between the foot and the ground during running is typically 2-3x body weight, whereas the peak forces experienced by the shin (lower leg) bone are typically 6-14x body weight! As such, these wearable accelerometer metrics may not provide a complete representation of the loading experienced by a runner’s bones, muscles, and tendons.
More research is needed to understand the utility of running accelerometer wearables for understanding or predicting running injury risk and resulting running performance. Would these running impact metrics have gained popularity if it weren’t for the growth of small, wireless, inexpensive, and convenient to implement accelerometer and IMU hardware? Of note, hardware availability wasn’t the only motivator, as misapplications of running impacts as an indicator of injury risk was pervasive in lab-based academic research well before the explosion of wearables [7]! However, it is plausible that hardware convenience stimulated at least some of the misapplications or misinterpretations.
“Colleagues often ask me if they should use a certain wearable device for elevating sports performance. I try to explain that it’s not about the device itself, it’s about why they want to use it. Too often, there is no ‘why’ driving the desire to use a device, just the fact that it’s available and interesting. I think this trips up many practitioners and leads to poor outcomes.” says Jessica Zendler, PhD, Special Consultant, Applied Safety and Ergonomics, Inc., a Rimkus Company during an interview for this article
In summary: First, miniaturized accelerometer and IMU hardware became available and convenient to integrate into sports settings (hardware availability). Next, wearable companies adopted this hardware and identified sensor signals or features of the sensor signals that could readily be reported to the user (not necessarily a signal of interest). Finally, these convenient to integrate sensors became widely adopted by athletes and used as a tool for quantifying running workload or injury risk. The integration of wearable accelerometers into sports performance monitoring seems, in some contexts, to have unfortunately been motivated by hardware availability, rather than by a signal of interest.
Let’s drive this point home: think back to the last time you were hungry and ordered food delivery or went out to grab a bite. When I’m having those Friday night food delivery cravings, I rarely select the most convenient restaurant or the one with the fastest delivery time (thank you to all those Uber Eats drivers who drive my food across town). Rather, I identify the type of food I am craving (99% of the time its super spicy Indian food), and then I select a restaurant that provides that food. Satisfy your athletes cravings by providing them with the wearable signals of interest that will elevate their performance!
#2: Valuable wearable signals are intuitive and actionable, but are also supported by meaningful target benchmarks.
Spatiotemporal metrics, such as step count, distance traveled, running speed, or cadence, reported by wearable activity monitors are easy to understand and intuitive for an athlete to act on. For example, if a runner sees that their current lap speed is lower than the target for the workout, they will try to increase their pace. Likewise, the number of steps an individual takes per minute (cadence) is readily understood by the athlete. The downfall of many of these measures, however, is knowing when to act on them. Often, there are no well-established target values of wearable measurements. The lack of meaningful benchmarks is partially because wearable measurements have not always been linked to performance outcomes. Prospective researcher studies in which wearable measurements and performance outcomes are synchronously tracked may help elucidate benchmarks that indicate a change in performance capabilities, but such studies are logistically challenging (expensive, time-consuming, etc.). Moreover, the resulting datasets from prospective studies can often be quite messy, with inconsistent trends between individual athletes. Just like every athlete is unique, a single threshold for action or optimal performance outcomes (e.g., 10,000 steps per day) is unlikely to fit every athlete. For example, a study of professional ultramarathoners found that cadence varied among athletes from 150 to over 200 steps per min [9], suggesting that a blanket prescription for a running cadence of 180 or higher is misguided.
Wearables that monitor spatiotemporal metrics provide an example of devices that are intuitive (what) and actionable (how), but such metrics are not always supported by meaningful target values to indicate when and to what extent behavior changes should be made. For now, the use case of many wearables is to monitor trends within an individual athlete through day-to-day monitoring, rather than relying on globally accepted benchmark measures. For example, some physiology researchers propose understanding an athlete’s specific heart rate variability “fingerprint” before using it as an indicator of training status [10]. Cycling power is another example of a “wearable” metric (power meter sensors are typically on the bicycle, not the body) that is intuitive and actionable, but target power values must be determined on an athlete-specific basis through observation over time [11]. Let’s accept that universal meaningful benchmarks for wearable-based measures are rarely known and likely an unrealistic expectation. Instead, let’s work to understand how wearable-based metrics trend within an individual athlete, allowing meaningful and personalized training changes. Sports scientists would benefit from patiently integrating wearables into their performance monitoring routines and allowing time to evaluate trends in these measures over time. Our understanding of meaningful benchmark measures and the tools we use to monitor these factors will progress in parallel over time.
“It’s easy to be overwhelmed by the amount of data that high-tech wearable sensors produce. Helping athletes figure out which metrics and trends are worth paying attention to is where sport scientists can add great value. A big part of this is layering in each individual athlete’s context (their goals, circumstances, etc.) to help them make meaningful and personalized training and performance decisions,” says Sian Allen, PhD, lululemon Product Innovation team
Let’s drive the point home: The earliest land surveying tools may have not been the most accurate and calibrated tools for measuring distance and elevation, but they helped create extraordinarily reasonable early maps of the earth’s land surface. Advancements in surveying tools certainly helped refine land maps, but the early integration of tools were crucial for building awareness of the Earth’s relative topography. We don’t need perfectly calibrated tools and target benchmarks in order to start mapping out an athlete’s performance capabilities.
#3: Fusing multiple wearable sensor signals into summary metrics is a promising approach for capturing the web of biomechanical, physiological, and cognitive factors that influence sports performance
My PhD dissertation focused on using wearable sensors to monitor two very specific types of overuse injuries: tibial stress fractures in runners and low back overexertion injuries in individuals that perform repetitive manual lifting. If I had a penny for every enthusiastic researcher, sports scientist, or wearable device developer that asked me “Can we use wearables to predict OVERALL injury risk?”, I’d be a happy retired 28-year-old. Unfortunately, the expectation that a single wearable sensor signal could predict the risk of injury to every muscle, bone, tendon, or other musculoskeletal tissue as well as capture every factor that contributes to injury risk is probably unrealistic. Rather, multiple wearable sensor signals may need to be fused (combined) to estimate a signal of interest. Since I cannot retire just yet, at least these exciting opportunities will hopefully keep me employed for many years to come.
“There is great enthusiasm from the sports science community for concise, easy to digest wearable summary metrics or summary reports. However, such summary metrics should be adopted with caution prior to fundamental research evaluating their association to sports performance” says Kristof De Mey, Sports Technology, Innovation & Business developer at Ghent University
Fusing multiple wearable sensor signals means combining numerous wearable metrics together with (often proprietary) algorithms to yield a summary metric. The individual metrics could come from multiple sensors distributed across the body or from multiple sensors embedded into a single device. For example, the current Oura Ring is outfitted with seven temperature sensors, a motion sensor, and LED sensors. An example of this sensor fusion approach is Apple’s new walking steadiness metric, which fuses numerous mobility metrics (walking speed, step length, double support time, and walking asymmetry, etc.) into one overall score. As another example, research shows that a combination of biosignals (heart rate, heart rate variability, respiratory rate, temperature, and activity) from an Oura Ring could detect signals associated with the onset of COVID-19 [12]. These summary metrics may also integrate non-sensor measurements, such as subjective ratings of fatigue, stress, pain, or other cognitive factors. An advantage of these summary metrics are they fuse multiple biomechanical, physiological, and cognitive factors into one easy to evaluate, holistic sports performance (or health, wellness, etc.) score. Mapping out athlete’s capabilities will require measurements of multiple factors that influence performance, rather than only isolated measurements.
A disadvantage of these new and emerging summary metrics is that they are inherently new and emerging, and there is not always an established measure to validate the accuracy of these measures against. For example, there is no medical guideline for “walking steadiness” or an obvious ground-truth measure, so it is unclear how one would validate its accuracy or reliability. In the absence of clear validation procedures, sports scientists can benchmark emerging summary metrics against expectations before adopting any new and seemingly intuitive summary metrics. For example, the seemingly intuitive acute to chronic workload ratio [13], which fused short-term and long-term training measures, was widely adopted (internationally!) as an indicator of training load and overall injury risk. Unfortunately, this summary metric was later identified as being a statistical artefact with no association to injury risk [14]. While this summary metric did not explicitly rely on wearable sensor data, it provides an example of how careful inspection can reveal a spurious and not meaningful association between wearable summary metrics and relevant outcomes [15].
Let’s drive the point home: Have you ever woken up in the middle of a winter night freezing because you accidently kicked the bedroom’s air vent to the closed position before heading to sleep? I remember groggily stumbling to the thermostat on the other side of my apartment confused at its reading of a toasty 70 degrees Fahrenheit. My apartment’s thermostat gives me information about the temperature in the room where my thermostat and temperature sensor are located, but doesn’t provide accurate information about the temperature in other areas. Smart thermostats or smart air vents fuse data from multiple sensors across your house to more accurately predict the temperature in each and every room. Outfit your athletes will wearables that provide a variety of measurements and a holistic picture of their sports performance.
Concluding remarks
Wearables for monitoring sports performance is still a new and emerging field, and we can expect ongoing innovation in the coming years. These three considerations can guide the integration of wearable today, and also provide hints of where there are future opportunities for improvement and innovation.
Agree or disagree with these considerations? Have a different take or other key learning suggestions? Please comment below or join the Twitter discussion.
---------------------------------------------------------------------------------
About the author
Emily Matijevich is currently a postdoctoral researcher at the University of Calgary in Alberta, Canada. She is passionate about leveraging biomechanics, wearables, and data science to invent and evaluate technologies that monitor human movement, improve mobility, and elevate performance. In addition to academic research, Emily has engaged in biomechanics and algorithm development research at numerous sports and wearable technology companies (e.g., Brooks Running, CCM Hockey, Orpyx Medical Technologies). Learn more about Emily here.
References
[1] M. AlGhatrif and J. Lindsay, “A brief review: history to understand fundamentals of electrocardiography,” J. Community Hosp. Intern. Med. Perspect., vol. 2, no. 1, p. 10.3402/jchimp.v2i1.14383, Apr. 2012, doi: 10.3402/jchimp.v2i1.14383.
[2] J. Achten and A. E. Jeukendrup, “Heart Rate Monitoring,” Sports Med., vol. 33, no. 7, pp. 517–538, Jun. 2003, doi: 10.2165/00007256-200333070-00004.
[3] R. W. Willy, “Innovations and pitfalls in the use of wearable devices in the prevention and rehabilitation of running related injuries,” Phys. Ther. Sport, vol. 29, pp. 26–33, Jan. 2018, doi: 10.1016/j.ptsp.2017.10.003.
[4] C. Neuwirth, C. Snyder, W. Kremser, R. Brunauer, H. Holzer, and T. Stöggl, “Classification of Alpine Skiing Styles Using GNSS and Inertial Measurement Units,” Sensors, vol. 20, no. 15, Art. no. 15, Jan. 2020, doi: 10.3390/s20154232.
[5] A. Alanen, A. Räisänen, L. Benson, and K. Pasanen, “The use of inertial measurement units for analyzing change of direction movement in sports: A scoping review,” Int. J. Sports Sci. Coach., vol. 16, no. 6, pp. 1332–1353, Dec. 2021, doi: 10.1177/17479541211003064.
[6] J. M. Peake, G. Kerr, and J. P. Sullivan, “A Critical Review of Consumer Wearables, Mobile Applications, and Equipment for Providing Biofeedback, Monitoring Stress, and Sleep in Physically Active Populations,” Front. Physiol., vol. 9, 2018, doi: 10.3389/fphys.2018.00743.
[7] E. S. Matijevich, L. M. Branscombe, L. R. Scott, and K. E. Zelik, “Ground reaction force metrics are not strongly correlated with tibial bone load when running across speeds and slopes: Implications for science, sport and wearable tech,” PLOS ONE, vol. 14, no. 1, p. e0210000, Jan. 2019, doi: 10.1371/journal.pone.0210000.
[8] W. B. Edwards, “Modeling Overuse Injuries in Sport as a Mechanical Fatigue Phenomenon.,” Exerc. Sport Sci. Rev., Jul. 2018, doi: 10.1249/JES.0000000000000163.
[9] G. T. Burns, J. M. Zendler, and R. F. Zernicke, “Step frequency patterns of elite ultramarathon runners during a 100-km road race,” J. Appl. Physiol., vol. 126, no. 2, pp. 462–468, Feb. 2019, doi: 10.1152/japplphysiol.00374.2018.
[10] D. J. Plews, P. B. Laursen, J. Stanley, A. E. Kilding, and M. Buchheit, “Training Adaptation and Heart Rate Variability in Elite Endurance Athletes: Opening the Door to Effective Monitoring,” Sports Med., vol. 43, no. 9, pp. 773–781, Sep. 2013, doi: 10.1007/s40279-013-0071-8.
[11] S. A. Jobson, L. Passfield, G. Atkinson, G. Barton, and P. Scarf, “The Analysis and Utilization of Cycling Training Data,” Sports Med., vol. 39, no. 10, pp. 833–844, Oct. 2009, doi: 10.2165/11317840-000000000-00000.
[12] A. E. Mason et al., “Detection of COVID-19 using multimodal data from a wearable device: results from the first TemPredict Study,” Sci. Rep., vol. 12, no. 1, Art. no. 1, Mar. 2022, doi: 10.1038/s41598-022-07314-0.
[13] B. T. Hulin, T. J. Gabbett, D. W. Lawson, P. Caputi, and J. A. Sampson, “The acute:chronic workload ratio predicts injury: high chronic workload may decrease injury risk in elite rugby league players,” Br. J. Sports Med., vol. 50, no. 4, pp. 231–236, Feb. 2016, doi: 10.1136/bjsports-2015-094817.
[14] F. Impellizzeri, S. Woodcock, A. J. Coutts, M. Fanchini, A. McCall, and A. Vigotsky, “Acute to random workload ratio is ‘as’ associated with injury as acute to actual chronic workload ratio: time to dismiss ACWR and its components.” SportRxiv, Mar. 03, 2020. doi: 10.31236/osf.io/e8kt4.
[15] L. Lolli et al., “The acute-to-chronic workload ratio: an inaccurate scaling index for an unnecessary normalisation process?,” Br. J. Sports Med., vol. 53, no. 24, pp. 1510–1512, Dec. 2019, doi: 10.1136/bjsports-2017-098884.
Comments
There are no comments yet.